Machine Learning vs. AI: Definitions, Differences & Application
Last Updated March 27, 2024
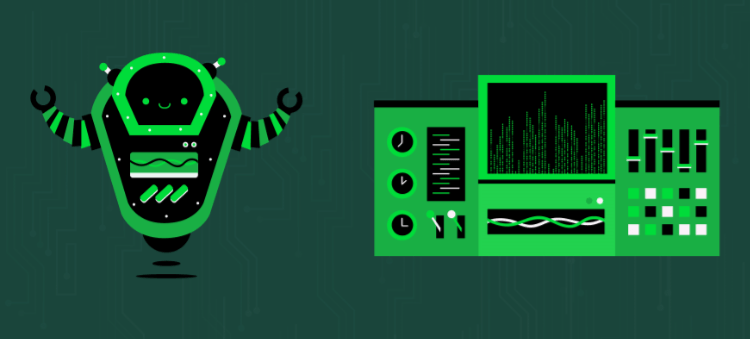
An explosion of advancement in the application of electronic brains, often housed under the umbrella term “Artificial Intelligence,” has many professionals striving to navigate a new frontier where machine learning and cognitive computing enable intelligent machines to reason and analyze like humans.
“We live in an enabled world, so how do human beings fit into this world of AI and machine learning?” says Keith Niblett, Assistant Director of Executive Development Programs at Michigan State University’s Eli Broad College of Business.
While the applications and capabilities of artificial intelligence and machine learning are rapidly evolving with “new” uses disrupting entire industries overnight—from building predictive algorithms for marketing trends and performance, to AI-powered demand forecasting within the supply chain, to transforming the employee hiring process—the programming behind them is not new.
The 1956 Dartmouth Summer Research Project on Artificial Intelligence effectively launched the field of AI, and IBM researcher Arthur Lee Samuels developed one of the first machine learning programs, coining the term in a 1959 research paper. But it’s the recent advancements in modern processor speeds, open-source algorithms, and data storage, availability, and improved visualization that has helped to launch AI and machine learning into broader applications across multiple industries.
As humans continue to push the boundaries of these capabilities and wrestle with their impacts and ramifications, understanding the best uses and applications of these intelligent machines is critical. One important distinction is that machine learning and artificial intelligence are not interchangeable functions; despite clear overlap between the two capabilities, there are also clear differences.
What is Artificial Intelligence?
Simply put, artificial intelligence is the science and technology of making machines, especially computer programs, intelligent, with that “intelligence” being the computational ability of these “programs to solve problems and achieve goals in the world as well as humans,” as explained by computer scientist John McCarthy, the “Father of AI” and one of the organizers of the 1956 Dartmouth Conference.
With this computational intelligence, AI machines aren’t programmed to do one single, repeated motion; instead, they adapt to situations and perform tasks in intelligent ways. AI machines are typically specialized, such as automated vehicle or smart stock trading systems. This is referred to as narrow AI. Researchers hope a future form of general AI may accommodate multiple tasks carried out in smarter ways—more like a human.
AI can be applied to predict when machines need maintenance or analyze large-scale systems or processes (for example, a factory’s end-to-end manufacturing process) to improve efficiency. AI also has applications in the service industry, like the “Just Walk Out” technology employed by Amazon to power its cashier-less Amazon Go stores. The freight arriving at those stores may soon be delivered by driver-less trucks powered by automated vehicle technology, with the potential to replace 2 million American truck drivers.
What is Machine Learning?
Machine learning is one of four main subsets of AI (machine reasoning, automated planning and natural language processing being the other three) and has the dynamic ability to “learn” from data without being programmed to do so. In other words, this is artificial intelligence without the complex codes and decision trees required for programming that AI cannot learn. The key to “training” the machine’s intelligence to think logically and understand with a “human-like” capacity is through massive amounts of data fed through an algorithm that adjusts and improves. For example, if recognition is a key application, over time, machine learning can hone its facial recognition to consistently recognize an individual’s face, even if that person has changed hairstyles, clothes or added someone else to the picture.
While AI has greatly impacted task-oriented jobs in the manufacturing and service industries, knowledge-based tasks and white-collar jobs are also feeling the effects of intelligent machine learning. Look no further than Alexa, Amazon’s ubiquitous voice assistant which continues to get closer to functioning like a human assistant through “active learning, in which the system identifies areas it needs help from a human expert,” and “self-learning,” where the system is “learning from implicit feedback,” automatically making corrections based on contextual clues from its human interactions, explains Rohit Prasad, vice president and chief scientist of Amazon Alexa. This kind of deep learning mirrors the human brain, using Artificial Neural Networks (ANN) in layers, each responsible for one facet of “learning.”
Differentiating Artificial Intelligence from Machine Learning
Ultimately, artificial intelligence and machine learning are complementary: Machine learning improves AI by accelerating the learning and increasing its sophistication. The artificial intelligence powered by this machine intelligence makes all this learning worthwhile, packaging the depth of knowledge in a useful application.
Applying Artificial Intelligence and Machine Learning
The key to effective application is in understanding the areas where artificial intelligence and machine learning applications can boost an organization’s business, and that can mean differentiating between the two based on the business problem to solve. Will the application need ongoing learning, such as predicting customer purchasing behaviors? If so, machine learning may be required to constantly learn from the data and remain up to date. If the need is for efficient customer data processing, a simpler form of AI may fulfill this.
Artificial intelligence and machine learning will only continue to advance, and organizations need to recognize which specialized skillsets will be needed in their employees to maximize this technology for the benefit of people—inside and outside of the organization.