Analytics in College Hoops: A New Type of March Madness
Last Updated March 28, 2024
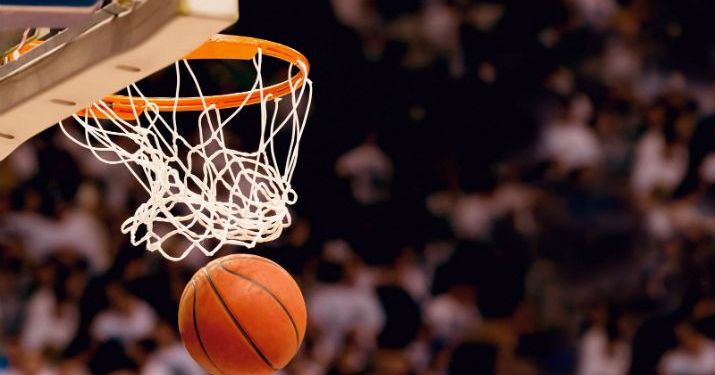
Statistics have always played a big role in sports, serving as a barometer for success rates for players and teams as a whole. In recent years, the development of analytic measurements has affected every aspect of sports, from game day coaching decisions to the definition of what makes a player the right fit for a specific team.
In basketball, analytics has become a driving force in the philosophy of how players are recruited, built and managed, going beyond examining statistics and positions to understanding how the pieces of a team fit together. Player traits, such as the amount of running during a game, effectiveness with the ball in possession, shooting position on the floor or the direction they are most likely to go when dribbling is analyzed to find which players complement each other best in an effort to maximize team chemistry (how players interact with each other) and achieve results.
For example, rather than deeming a player a point guard or power forward, based on size and ability to fulfill the typical attributes related to those positions, coaches now look at players in terms such as “scoring rebounder,” a player that can score and retrieve the ball after missed shots, or “paint protector,” a player able to block shots in the painted area near the basket.
This expanded thinking helps when it comes to strategy and game planning. Personnel packages can be tailored by which players enjoy the best chemistry rather than simply pinning everything on athletic ability.
Additionally, teams or specific personnel packages can be measured in statistical terms meant to apply to the group as a whole rather than an individual player. Where simple field goal percentage and points allowed was once considered enough to tell the tale of a team’s success, now analysts with laptops stationed behind team benches are looking at advanced metrics like points per possession and adjusted defensive efficiency to get a more complete grasp of how teams are performing.
The value of analytics can be extended to other collegiate sports such as hockey. Examining defensive pairings or player compatibility can influence decisions to assign a player position that could impact the game.
Analytics in College Basketball
If coaches want to analyze the effectiveness of their players, there are plenty of ways to do it. To keep up with this changing tide, Michigan State basketball took its own approach.
Kevin Pauga, Michigan State’s former Director of Basketball Operations and current Assistant Athletic Director of Administration, is considered a leading thinker in the analytics field. Having developed his own system, known as the Kevin Pauga Index (KPI), for ranking college basketball teams, Pauga has been able to accurately predict the field of the NCAA Tournament in years past.
Using Microsoft Excel, Pauga built a custom program that places a value on each game played during a season for any team in college basketball. The program plugs data into an algorithm Pauga has created which takes into consideration an array of factors, from the pace of play to the time zone in which the game is played. The algorithm has even been used by the NCAA Tournament selection committee due to the diversity of metrics and the ways it can sort team statistics. It is a constant work in progress, as Pauga recently fine-tuned the algorithm to assign the value to a home, neutral venue or road victories.
The MSU alum started as a student manager for the Spartans basketball team where he began charting statistics such as “defensive intensity,” a measurement of how often a player touches the ball on defense. KPI is now as widely recognized by college basketball analysts as the RPI (Rating Percentage Index) developed by the NCAA in 1981.
Analytics in the NBA
The use of analytics in college basketball is not nearly as developed as the NBA, as the college level is considered a developing ground for players and the technology surrounding this data is still in its infancy.
Professional basketball has seen the adoption of analytics across the board, with some general managers such as Daryl Morey of the Houston Rockets prioritizing analytics data over traditional ways of thinking about basketball. Morey has become known as a poster boy of sorts for analytics, his successes and failures held up as evidence that analytics either do or don’t work. The argument is heated among media pundits, but less so in NBA front offices where data plays a major role in decision making.
In recent years, the NBA has gone so far as to modify arenas with six player-tracking cameras in every stadium league-wide. The technology, called SportVU, records every movement a player makes during the game. Between the six cameras, they can capture the movement of 11 designated objects (10 players and the ball).
Basketball systems analyst Peter Beshai developed a website that tracks key statistics of some of the NBA’s most prolific players to give an example of what the analytics data actually looks like. Charts display shot frequency and field goal percentage by distance and location on the court. Graphs can be used to show a player’s effectiveness against certain teams.
Opponents can look at this data and see that NBA star Kevin Durant, for example, prefers to shoot from the right side of the court when shooting from a three-point range and his field goal percentage from beyond the arc is actually at its lowest when he is closer to the three-point line. In theory, this could affect the approach teams take when defending Durant.
As technology and data-gathering continue to evolve, analytics will continue to drive player and play-making decisions, from the front office to the court.